Real-time CO2 emission estimation using FootNet
We demonstrate a computationally efficient GHG flux inversion framework using a machine learning emulator for atmospheric transport (FootNet) as a surrogate for the full-physics model. The measurement footprints generated by FootNet are at approximately one-kilometer resolution. This flux inversion using a machine learning surrogate model only requires meteorological data, GHG measurements, and prior fluxes and is completely independent of full physics atmospheric transport models. The 650x speedup allows for computation of footprints “on-the-fly” during the GHG flux inversion (i.e., computed as needed, rather than archiving for future use) and makes real-time emission monitoring computationally possible. This work alleviates a major computational bottleneck with inferring GHG fluxes with next generation dense observing systems.
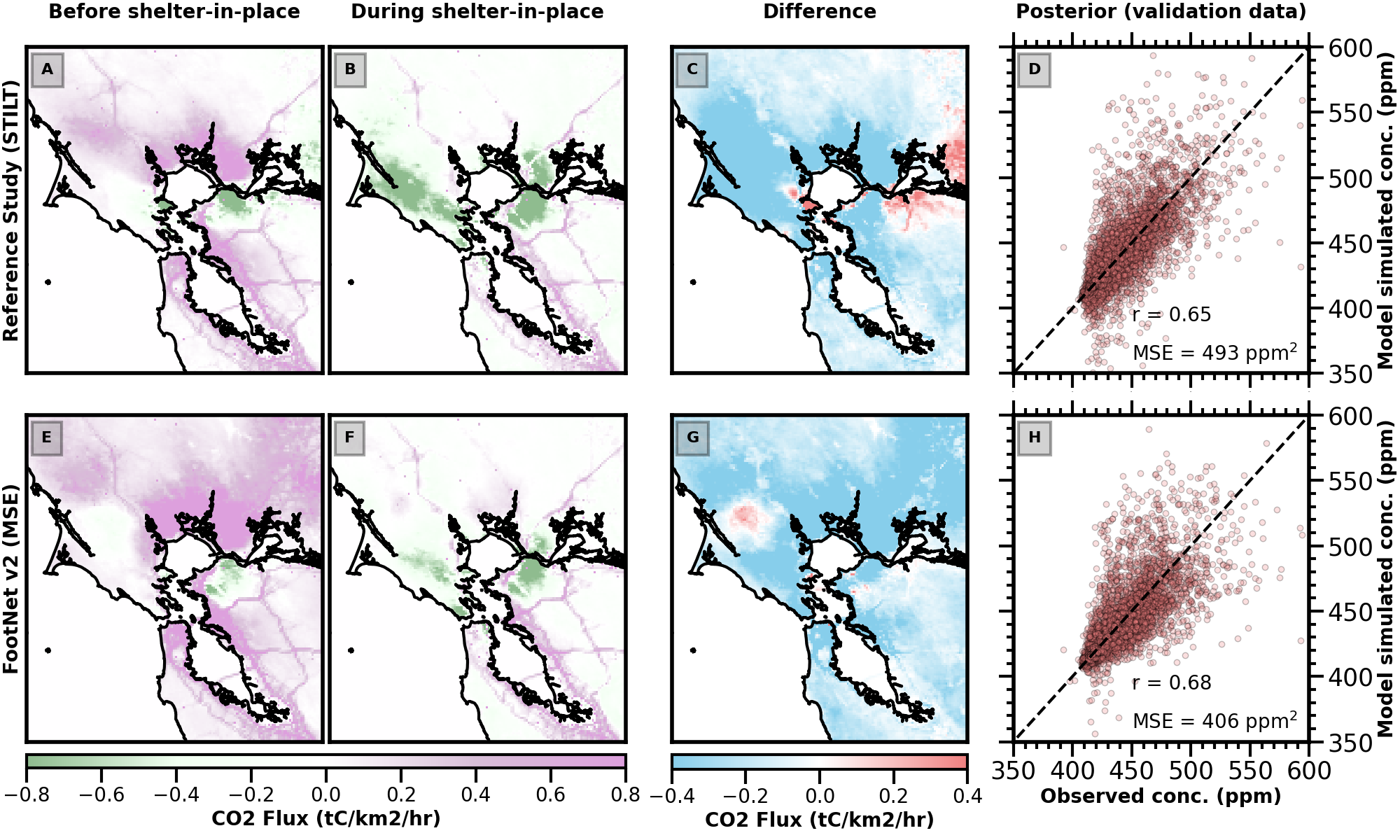
Fig. The comparison of GHG emission source estimation between FootNet and full physics atmospheric transport model (STILT) for San Francisco Bay area before and during COVID-19.Citations
Dadheech, N.*, He, T. L.*, & Turner, A. J. (2024). High-resolution greenhouse gas flux inversions using a machine learning surrogate model for atmospheric transport. EGUsphere, 2024, 1-21. in review, * equally contributed authors. Download PDF