FootNet: Machine Learning Emulator for Atmospheric Transport
Full physics atmospheric transport models (e.g. STILT, Flexpart etc.) are computationally expensive as well as storage intensive for high-resolution dense observing systems. Here we developed a machine learning emulator for atmospheric transport (FootNet) which is based on U-Net model architecture. FootNet is trained on measurement footprint outputs from STILT simulations. FootNet computes a measurement 1000x faster than a full physics model and provides a 650x speed up in estimating GHG emission sources using flux inversion. Footprints can be computed on-the-fly with FootNet which allows for real-time GHG emission monitoring at high-resolution with dense observing systems.
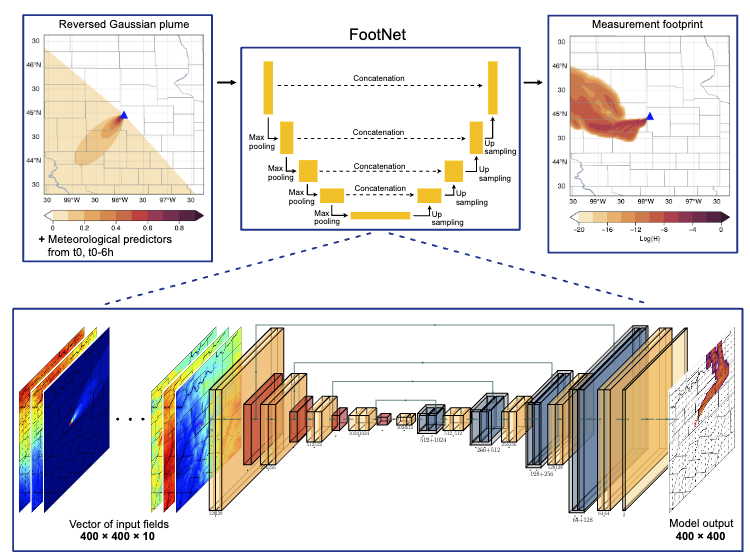
Fig. Top row shows the schematic diagram of the FootNet model. Detailed structure of FootNet is shown at the bottom.Citations
Dadheech, N., He, T. L., & Turner, A. J. FootNet v3: Generalized machine learning emulator for atmospheric transport for surface and space-borne observations (in prep).
Dadheech, N.*, He, T. L.*, & Turner, A. J. (2024). High-resolution greenhouse gas flux inversions using a machine learning surrogate model for atmospheric transport. EGUsphere, 2024, 1-21. in review, * equally contributed authors. Download PDF
He, T. L.*, Dadheech, N.*, Thompson, T. M., & Turner, A. J. (2023). FootNet: Development of a machine learning emulator of atmospheric transport. in review, * equally contributed authors. Download PDF