Research Projects
My research focuses on developing computationally efficient methods to identify greenhouse gas (GHG) emission sources using machine learning. I am currently using machine learning models to compute atmospheric transport in GHG flux estimation using Bayesian inference. I am also interested in detecting methane plumes (e.g. oil/gas basins) from space using Landsat 4 to 9 and Sentinel-2 satellites. Here are some of the projects that I have been working on:
FootNet model takes meteorology (e.g. winds, PBL height, surface pressure, Gaussian plume etc.) to compute source-receptor relationship (measurement footprint) for an observation. This is an important component of GHG flux inversion.
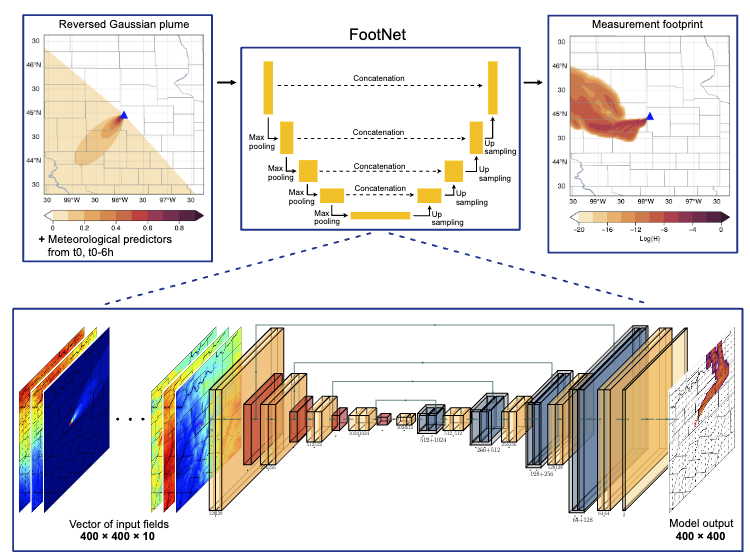
Here we developed a computationally efficient method for real-time estimation of GHG emission fluxes using a machine learning emulator for atmospheric transport (FootNet).
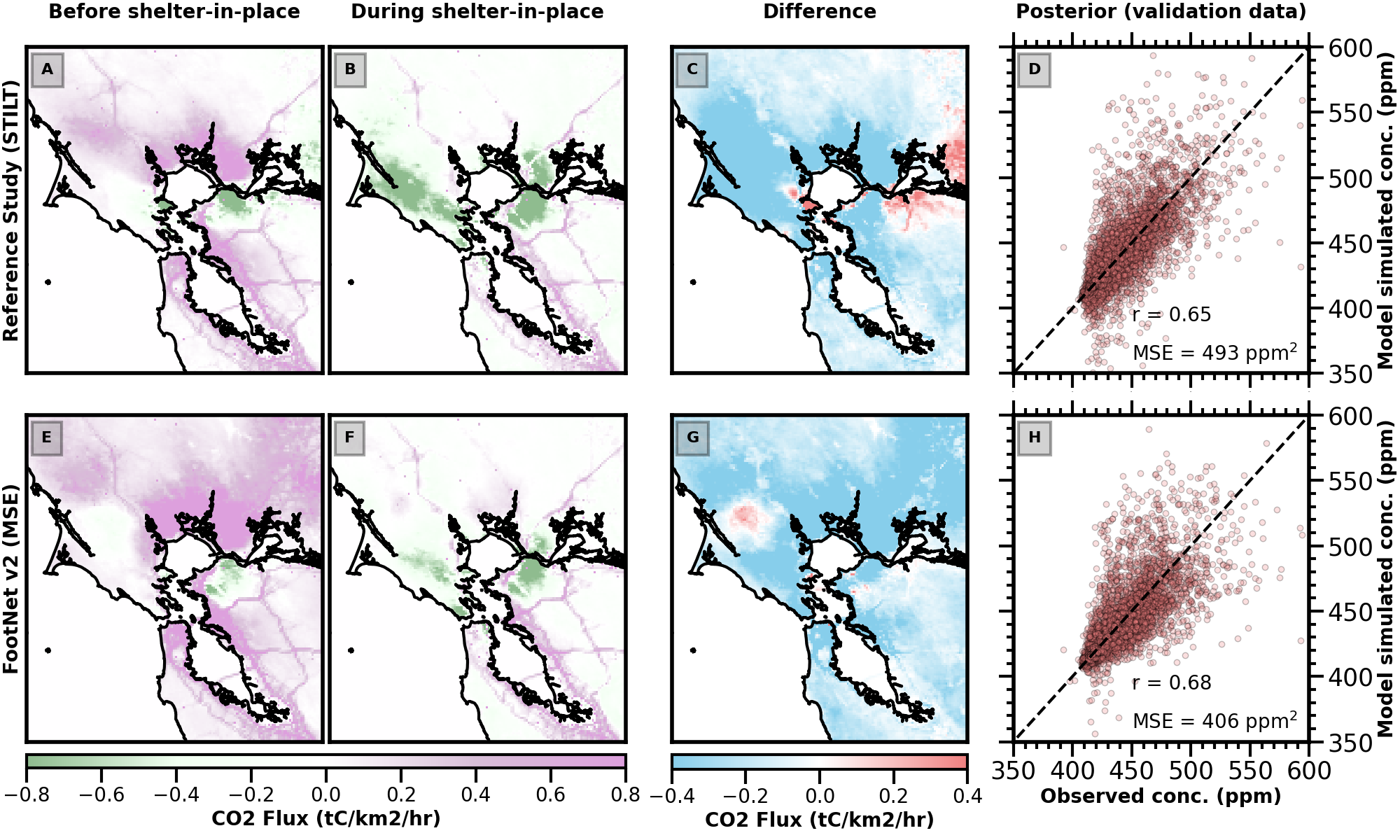